Hybrid Player Experience Model
Our objective is to increase positive experience of playing games by directed personalization. The key to achieve directed personalization is to develop a general model of motivation and fun. Based on this model we proceed with the analysis of player behavior that is represented by the data provided by the interaction of player and game. Non-invasive clustering of the behavioral data leads to patterns representing different play styles that can be analyzed and interpreted further [1]. The model of motivation and fun needs to be computer readable to infer information for personalization.
Our aim is to transfer these patterns to the player types introduced by Dr. Richard Bartle and the model of flow developed by M. Csíkszentmihályi.
The model of Bartle describes human personality in a game-playing context by introducing the four Bartle types [2]. These types differ from in each other in two main dimensions: player vs. world and acting vs. interaction. Each of the four Bartle types, namely Killer, Achiever, Socializer and Explorer, prefer an individual combination of these features. Each player is an individual mixture of all Bartle types, i.e. in general no pure identification as only one Bartle type occurs. Thus, every sphere of interest is covered.
- Killers: act on other players and affect the play experience of other players
- Achievers: act on the world by completing challenges of the game world
- Socializers: interact with other players and form relationships
- Explorers: explore the game world to gain a deeper understanding of the mechanisms
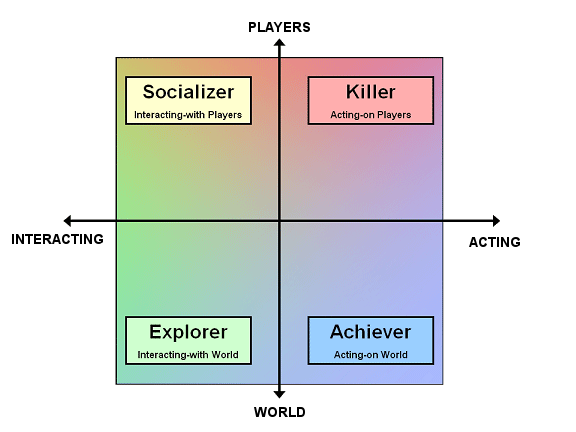
On the downside, Nicholas Yee points out that the Bartle types were not developed on the basis of empirical data and therefore, many types may overlap, as we mentioned earlier. Furthermore, the non-empirical development of these types may result in an improvable characterization which means that important traits are excluded and the types are built on unnecessary traits [3]. However, the Bartle types provide an understandable basis for our analysis. Additionally, we want to evaluate the Bartle types empirically on big data.
The second model we rely on to describe the player experience is the model of flow. M. Csíkszentmihályi developed the theory of flow which is referenced across a variety of activities [4]. Flow describes a mental state during an activity in which the person is fully involved in the process of the activity and is immerged in a feeling of happiness. In other words, flow is the perfect balance between ability and challenge and is therefore an individual experience of each player.
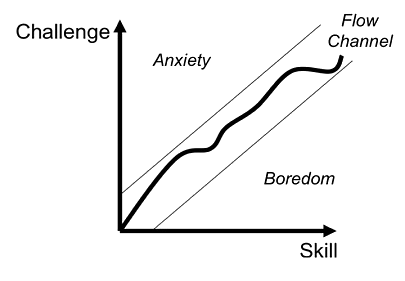
Smart analytics on big data by assessment of flow in our two-tier model enables the prediction of flow and thus personalized feedback for each player type and on individual level. The prevention of frustration and boredom by introducing personalized feedback is based on the detection of patterns leading to frustration and boredom on the one hand, and fun on the other hand. The machine learning methods to extract these patterns are based on huge amounts of behavior data.
Computational player experience modelling offers a promising possibility to analyze and improve artificial intelligence (AI) in games [6]. Hence we want to implement the player experience model by means of statistical models and AI-techniques and thus predict the loss of fun, analyze the cause of frustration/boredom on player type and individual level automatically in real-time. In this fashion our hybrid PEM can be applied in diverse real-world game environments ranging from online-games to gamified systems.
References:
[1] A. Drachen et al.: "Guns, Swords and Data: Clustering of Player Behavior in Computer Games in the Wild"[2] Bart Stewart: "Personality and play styles: a unified model"
[3] http://www.nickyee.com/facets/bartle.html
[4] M. Csíkszentmihályi: "Flow: the psychology of optimal experience"
[5] http://www.pbs.org/thisemotionallife/blogs/flow
[6] G. Yannakakis: "Game AI Revisited"